Topographical reconstructions from monocular optical colonoscopy images via deep learning
Mahmood F, Durr NJ
IEEE ISBIĀ 2018
https://ieeexplore.ieee.org/document/8363558
Abstract
Colorectal cancer is a leading cause of cancer deaths worldwide, but mortality can be mitigated by the detection and removal of premalignant lesions. Unfortunately, conventional 2D optical colonoscopy does not capture topographical information of the surface of the mucosa and thus has a high lesion miss rate. In this short paper, we use a joint deep convolutional neural network-conditional random field (CRF) framework for depth estimation from monocular colonoscopy images. Unlike previous approaches, this method does not make any geometric assumptions. The estimated depth is used to reconstruct the topography of the surface of the colon. Using digitally generated synthetic endoscopy data and CT-Phantom data, with corresponding ground truth depths, we train the unary and pairwise potential functions of a conditional random filed in a joint network. Results show that this approach can estimate depths for test data with an 84% accuracy. We show that estimated depth maps can be used for reconstructing the topography of the mucosa from real colonoscopy images. This topographical information can be used for improving learning-based algorithms for detection, segmentation and identification of lesions.
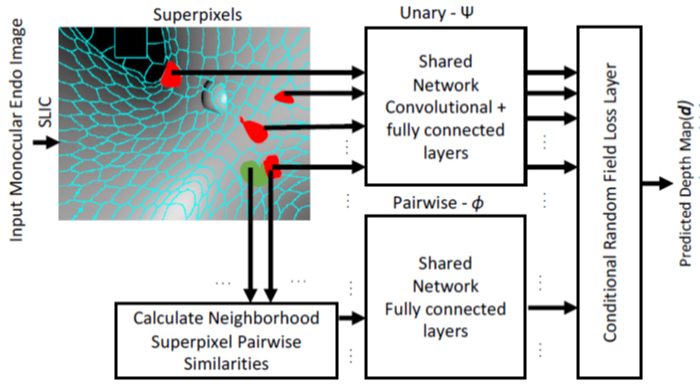