GAN inversion for data augmentation to improve colonoscopy lesion classification
Golhar M, Bobrow TL, Ngamruengphong S, Durr NJ.
IEEE Journal of Biomedical and Health Informatics 2024 [Journal Link] [Preprint]
Abstract
A major challenge in applying deep learning to medical imaging is the paucity of annotated data. This study explores the use of synthetic images for data augmentation to address the challenge of limited annotated data in colonoscopy lesion classification. We demonstrate that synthetic colonoscopy images generated by Generative Adversarial Network (GAN) inversion can be used as training data to improve polyp classification performance by deep learning models. We invert pairs of images with the same label to a semantically rich and disentangled latent space and manipulate latent representations to produce new synthetic images. These synthetic images maintain the same label as the input pairs. We perform image modality translation (style transfer) between white light and narrow-band imaging (NBI). We also generate realistic synthetic lesion images by interpolating between original training images to increase the variety of lesion shapes in the training dataset. Our experiments show that GAN inversion can produce multiple colonoscopy data augmentations that improve the downstream polyp classification performance by 2.7% in F1-score and 4.9% in sensitivity over other methods, including state-of-the-art data augmentation. Testing on unseen out-of-domain data also showcased an improvement of 2.9% in F1-score and 2.7% in sensitivity. This approach outperforms other colonoscopy data augmentation techniques and does not require re-training multiple generative models. It also effectively uses information from diverse public datasets, even those not specifically designed for the targeted downstream task, resulting in strong domain generalizability. Project code and model: https://github.com/DurrLab/GAN-Inversion .
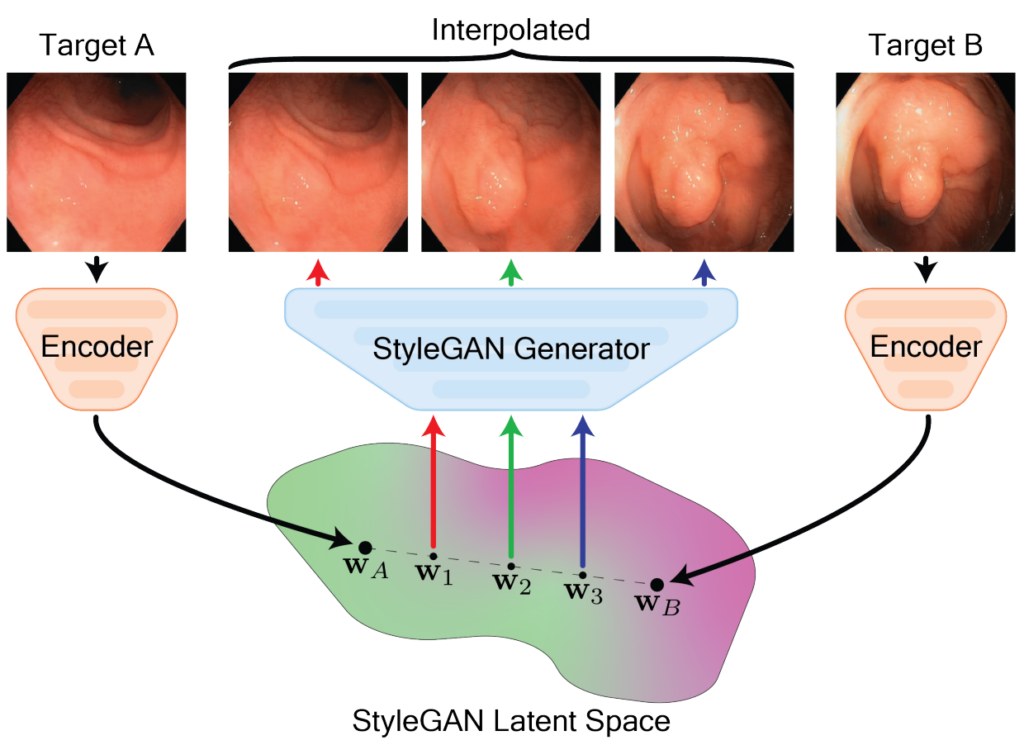